In the dynamic world of artificial intelligence and machine learning, transformer models have revolutionized the ways we understand and process language. Transforming language data into meaningful insights, these models are pivotal in the evolution of natural language processing (NLP). Among the numerous methodologies employed to test and refine these models, the TTR (Type-Token Ratio) test stands out as a significant indicator of linguistic complexity and versatility, especially when harnessed on transformer architectures. This article delves into the intricacies of applying the TTR test on transformer models and its profound implications for product development.
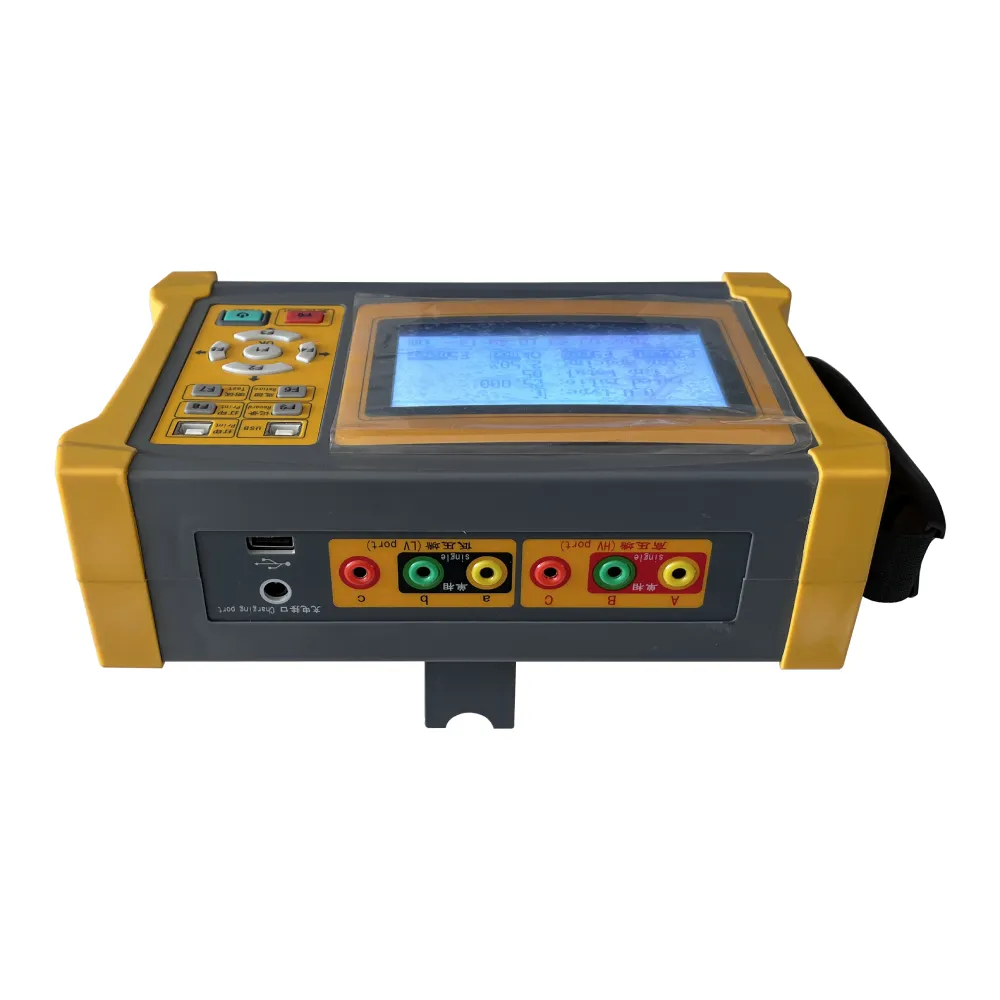
The essence of the TTR test lies in its ability to measure the diversity of vocabulary within a text. This linguistic test calculates the ratio of unique words (types) to the total number of words (tokens) in a given sample. A higher TTR indicates a richer, more varied vocabulary, which is essential for understanding and producing nuanced language constructs. When it comes to transformer models, which form the backbone of state-of-the-art NLP applications, evaluating them with the TTR test can yield insights into their ability to handle complex linguistic tasks.
Transformers have gained immense popularity due to their attention mechanisms, allowing them to contextualize words by focusing on different parts of input sequences. This characteristic is particularly beneficial when it comes to optimizing TTR on transformers, as it enables the model to discern and process intricate relationships between words. The TTR test, when applied to transformer models, helps ascertain their capacity to generate diverse and contextually pertinent responses, especially in content generation applications, chatbots, and virtual assistants.
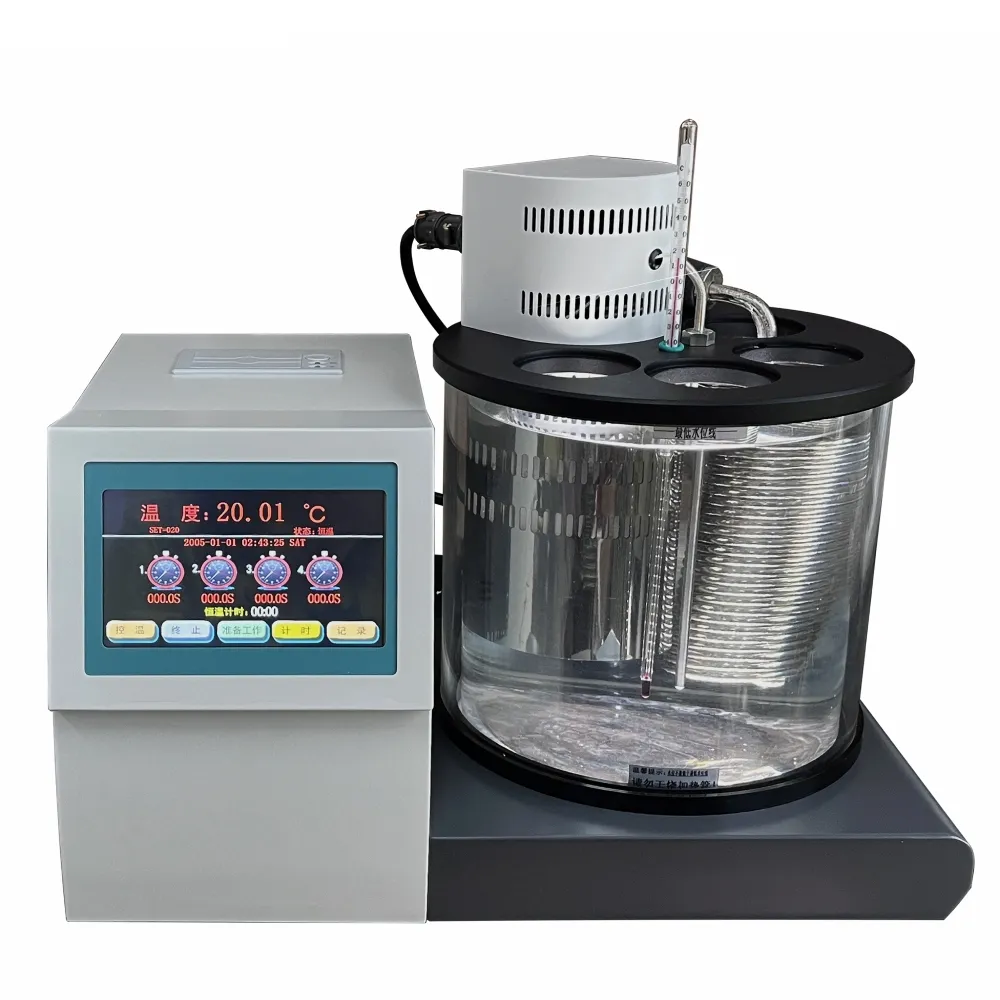
In practical applications,
the TTR test on transformers goes beyond mere academic interest. Companies developing linguistic products, such as advanced translation tools or language learning applications, can leverage TTR scores to enhance their offerings. By improving the ability of transformers to manage diverse language inputs, products can achieve higher levels of accuracy and user satisfaction. For instance, language learning apps that use transformer-based methods can track TTR scores to ensure learners are exposed to sufficiently diverse vocabulary, thereby improving their language acquisition process.
Moreover, the TTR evaluation on transformers aligns well with the principles of Experience, Expertise, Authoritativeness, and Trustworthiness (EEAT). From an experience perspective, the implementation of TTR tests ensures that products like interactive AI assistants provide end-users with responses that reflect genuine understanding and language adaptability. This enhances the user experience by maintaining engaging and relevant interactions.
ttr test on transformer
Expertise is reflected in the intricate design of transformers to evaluate TTR, showcasing a deep understanding of both linguistic properties and machine learning techniques. These models, backed by TTR analysis, demonstrate technical proficiency in dynamically adjusting to varied and complex language inputs, which is essential for maintaining product competitiveness.
Authoritativeness is established through consistent performance of transformer models in TTR evaluations, underscoring their reliability in producing linguistically varied and contextually sound communications. This aspect is crucial for users who depend on products that interpret and respond to language accurately.
Finally, trustworthiness is bolstered when TTR-enhanced transformer models deliver consistent, high-quality interactions. Trust is a cornerstone for users relying on AI-driven products, particularly in sectors where language precision and variability are paramount, like customer service automation or academic research tools.
In conclusion, testing transformer models with the TTR metric is not merely a theoretical exercise but a critical strategic tool that influences product quality and user satisfaction. For companies and developers, focusing on TTR in the context of transformers represents an opportunity to refine product capabilities, ensuring they resonate more effectively with diverse user bases. This approach positions products as leaders in their field, supported by sound linguistic analysis and robust machine learning methodologies. Therefore, embracing TTR tests in the development pipeline is not only wise but essential for technological innovation and consumer trust in AI-driven products.