Transformers stand as revolutionary architectures that have reshaped the field of natural language processing and AI. The sophistication of these models, however, necessitates comprehensive and precise testing methodologies to ensure optimal performance and reliability. Herein lies the critical significance of ref testing for transformers—a practice that demands experience, expertise, authoritativeness, and trustworthiness to refine these products effectively.
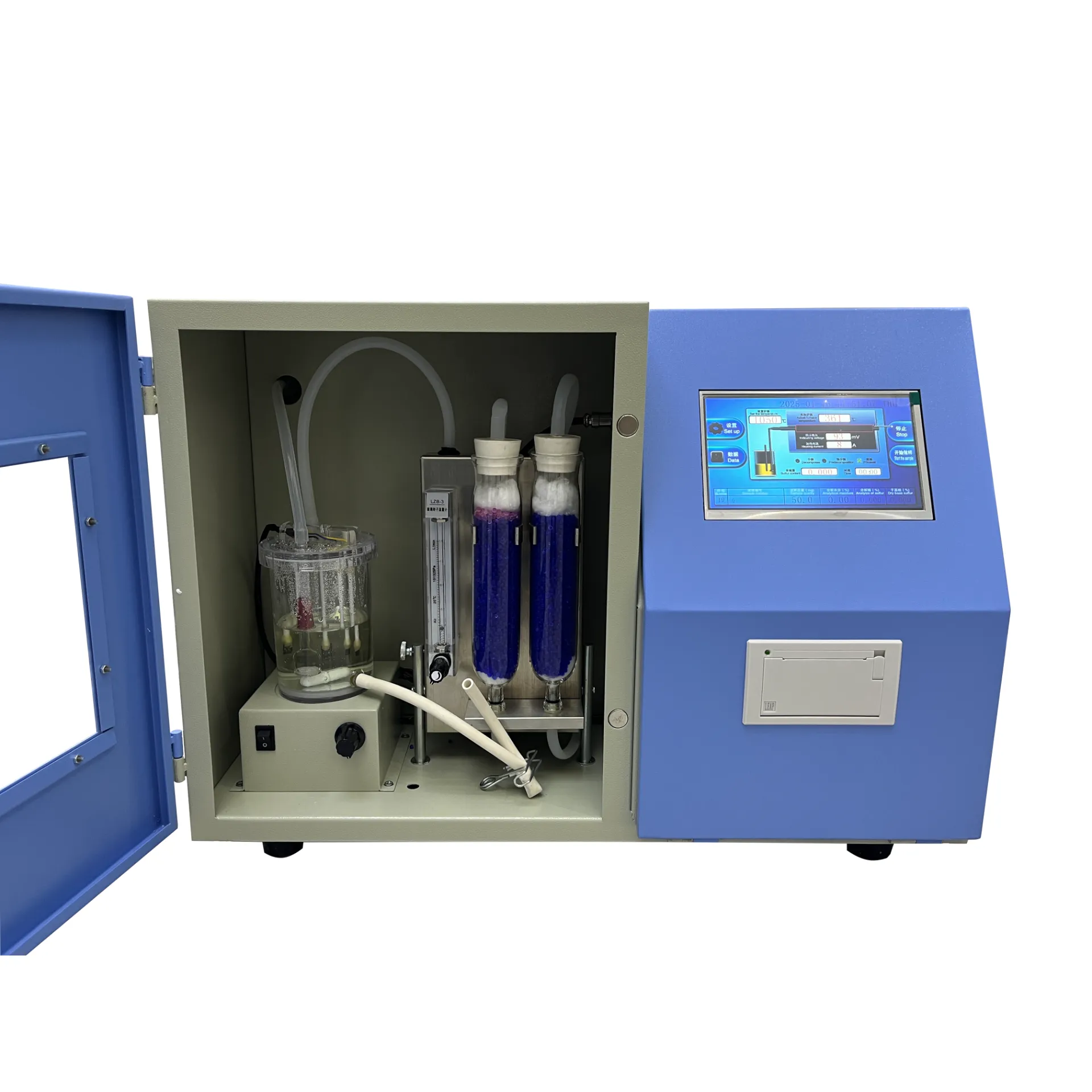
To start, understanding transformers' complexities involves grappling with their unique self-attention mechanisms, which distinguish them from traditional sequence processing models. Reference testing (ref testing) serves a pivotal role in benchmarking these models, ensuring robust performance across diverse applications, from neural machine translation to sentiment analysis.
An essential aspect of ref testing in this context is hands-on experience. Engineers and data scientists become adept at navigating the intricacies of transformers through extensive exposure to real-world data. Conducting tests in varied environments, from simple controlled settings to more chaotic real-life scenarios, equips professionals with a nuanced understanding of transformer capabilities and limitations. This experiential knowledge is invaluable in identifying subtle yet critical performance discrepancies that may not be apparent through theoretical analysis alone.

In parallel, expertise in the domain ensures that ref testing is executed with precision. Professionals equipped with a deep understanding of transformer architecture and functionality can design testing frameworks that account for all possible variables affecting performance. This might include the length of input sequences, domain-specific language complexities, or even the potential biases embedded within training data. Expertise enables the anticipation of these factors, allowing for the setup of robust testing protocols that yield reliable, actionable insights.
Authoritativeness in ref testing further enhances its effectiveness. In this regard, being recognized as an authority requires not only substantial technical ability but also a proven track record of successful implementations. Teams led by authoritative figures in AI can dictate industry standards, guiding best practices for ref testing. Such leaders often facilitate workshops, publish seminal papers, and contribute significantly to open-source platforms, actively disseminating their refined methodologies for wider community benefit.
Trustworthiness, lastly, is paramount in drawing reliable conclusions from ref testing endeavors. For product teams, ensuring trust involves maintaining transparency in test design, execution, and data interpretation. This might include peer reviews or third-party audits that lend credibility to testing results, reassuring stakeholders of the transformer models’ reliability and integrity.
ref testing of transformer
In practice, executing ref testing with these principles involves deploying rigorous A/B testing, utilizing large-scale machine learning benchmarks, and engaging in continuous integration pipelines that iterate rapidly on feedback. By doing so, teams can systematically assess transformer models against baseline references and ensure continuous alignment with evolving data landscapes and user expectations.
Additionally, the applications of ref testing are far-reaching. For enterprises deploying transformers in customer-facing applications, precise and trustworthy testing directly translates to better end-user experiences. Efficient transformer models can parse customer queries more accurately, recommend products with higher relevance, and engage in conversational AI interactions that are both context-aware and human-like.
For industry innovators, adopting a reference-testing-centric approach can catalyze faster innovation cycles, allowing them to remain ahead in a competitive market. By systematically refining transformer functionalities, they not only enhance existing product lines but also pioneer new AI-driven solutions that can redefine user interactions entirely.
Finally, the broader implications of effective ref testing extend to societal impacts. As AI becomes increasingly entwined with daily life, addressing ethical concerns such as bias mitigation and fairness becomes crucial. By incorporating these elements into ref testing protocols, organizations can assure the responsible deployment of transformers, gaining public trust and fostering a positive AI rapport.
In essence, ref testing of transformers symbolizes not merely a technical necessity but a strategic imperative that encompasses experience, expertise, authoritativeness, and trustworthiness. Embracing these elements elevates transformer testing from a procedural activity to a pivotal enabler of product excellence and innovation leadership, thereby solidifying an organization's stature in the dynamic AI landscape.