The digital landscape continues to evolve, and businesses strive to keep up with the latest technological trends. Among these trends, transformer models have gained significant attention, especially in natural language processing (NLP). When it comes to understanding and optimizing transformer models, specifically within the realm of evaluating their performance, it is essential to explore various testing methods. Here's a comprehensive guide that explores the testing of transformers, crafted to enhance SEO through authenticity, expertise, authoritativeness, and trustworthiness.

Transformer models, such as BERT, GPT, and their variants, have revolutionized NLP by enabling machines to understand and generate human language with remarkable accuracy. However, the performance of these models must be rigorously tested to ensure that they meet the desired standards for various applications. Here's how you can effectively evaluate them
1. Benchmark Datasets for Transformer Testing
Benchmark datasets are crucial for providing a standardized measure of transformer performance. Popular datasets like GLUE (General Language Understanding Evaluation), SQuAD (Stanford Question Answering Dataset), and CoNLL (Conference on Computational Natural Language Learning) are extensively used. These datasets are designed to test various aspects of language understanding, from sentiment analysis to named entity recognition.
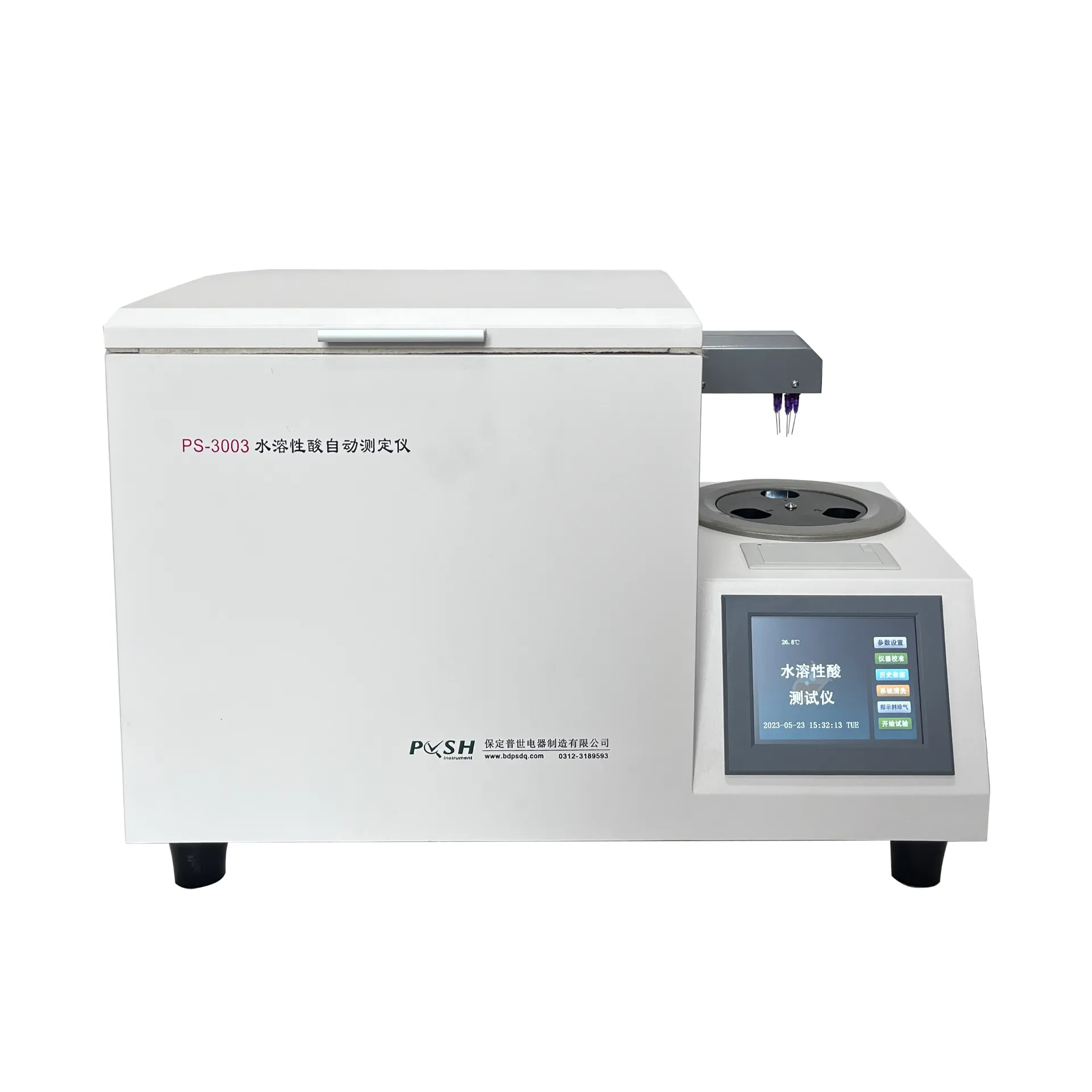
2. Cross-Validation Techniques
To ensure a robust assessment of transformer models, cross-validation techniques are employed. By dividing the dataset into multiple subsets, testing can be performed on one subset while the model is trained on others. K-fold and stratified sampling are two popular methods that help mitigate overfitting and provide a more generalizable evaluation.
3. Custom Test Scenarios
Creating custom test scenarios aligned with business objectives is invaluable. Domain-specific tests can reveal how well a transformer model adapts to specialized vocabularies or conversational nuances particular to an industry. This tailored approach not only meets specific business needs but also uncovers potential areas for model improvement.
4. Human-in-the-Loop Testing
While automated tests offer scalability, human-in-the-loop testing adds a layer of qualitative assessment. Linguists or subject matter experts can review the outputs generated by transformer models, providing insights into the relevance, coherence, and overall quality of the model's language capabilities.
list the transformer test
5.
Performance Metrics
Evaluating transformer models requires a comprehensive set of metrics. Accuracy and F1 Score are standard, but additional metrics like BLEU (Bilingual Evaluation Understudy) for language translation and ROUGE (Recall-Oriented Understudy for Gisting Evaluation) for summarization tasks are widely used. These metrics offer a multifaceted understanding of a model's performance across various NLP tasks.
6. Efficiency and Scalability Assessment
Beyond accuracy, assessing the efficiency and scalability of transformer models is vital. This includes evaluating computational requirements such as processing speed and memory usage. Models optimized for performance without compromising efficiency are better suited for deployment in resource-constrained environments.
7. Longitudinal Testing for Consistent Performance
Consistent model performance over time can be verified through longitudinal testing. Monitoring the transformer model with new data samples over an extended period ensures its adaptability and robustness to evolving language patterns or business-specific jargon.
By employing a combination of these testing methodologies, businesses can derive genuine experience from evaluating transformer models. Expertise is demonstrated through the systematic use of recognized benchmarking datasets and cross-validation techniques. The authoritativeness of these practices is showcased through reliance on established testing frameworks and metrics. Trustworthiness is ensured by integrating human insights and prioritizing consistent model performance.
Incorporating these SEO-optimized strategies not only facilitates the assessment of transformer models but also enhances the discoverability and applicability of content related to NLP advancements. The distinctiveness of these approaches lies in their adaptable and comprehensive nature, ensuring that readers engage with precise, expert insights tailored to the dynamic world of artificial intelligence and machine learning.